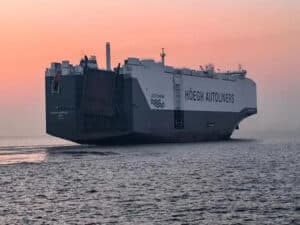
Op-Ed: How AI is making accurate CII predictions possible for shipping
Written by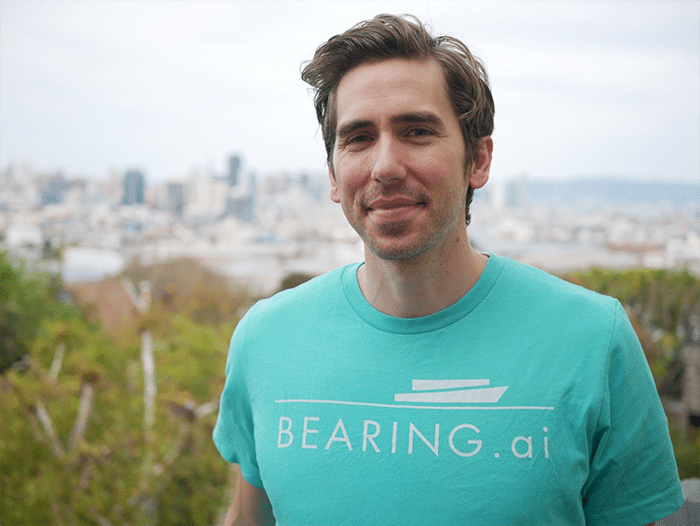
Dylan Keil, CEO Bearing
By Dylan Keil, CEO Bearing
The new year brought new goals for the world’s shipping fleet: Starting in 2023, the International Maritime Organization (IMO) required every vessel to receive a Carbon Intensity Indicator (CII) grade based on its emissions over the previous year.
Last year, Bearing compiled data from over 15,000 of the world’s shipping vessels to see how much of the global fleet was on course to receive passing ratings in 2023. According to 2022’s figures, 51.9% of vessels were slated to receive a failing rating of D or lower.
Since these ratings can have significant consequences for business operations, owners want to know how their ships will do. Predictive models can provide answers – but there are many kinds. What are the pros and cons of each?
Emissions models fall into two categories: physics-based and data-driven. Each tries to estimate fuel consumption — and so, emissions — over a given distance. But the ways they do this, and the accuracy of their results differ greatly.
Physics-based models use Newtonian mechanics to try to simulate real-world physical systems.
One such approach is scale modeling. This involves towing an actual scale-size ship model, typically about a meter or two long, through an experimental tank. This is sufficient to ascertain the hull’s resistance in varying water conditions and provide an estimate of real-world performance.
Another form is the semi-empirical formula. This is a calculation that combines the physical parameters of a ship, such as its dimensions, with real-world data about sea height and other conditions. With the aid of computational hydrodynamics, models based on these formulas can reach 80% accuracy.
Physics-based models have the advantage of being familiar and well-established, with examples dating back to the 1800s. Their limitations are rooted in human capacity. A deceptively simple factor like “wave height” contains within it innumerable dimensions including velocity, frequency, regularity and direction that no equation can account for. In addition, mathematically unpredictable factors like biofouling can make real-world results diverge dramatically from predictions.
That’s where the new generation of data-driven models comes in. These use artificial intelligence to tackle what physics-based models cannot. Instead of trying to model physical forces on a particular ship, data-driven models ingest a vast range of historic data about vessels and their voyages, and combine them with data about weather and sea conditions to predict future vessel performance.
For instance, an AI-powered model might ingest data about a ship’s RPM at a given moment, and the force and direction of the winds and waves, and hundreds of other factors besides. It doesn’t try to account for how forces interact. It learns from the aggregate data how the ship tends to behave. From there it can make predictions about how it will behave in the future.
This approach is only a few years old, but the results are remarkable. Based solely on localized weather data and publicly available AIS information for the global fleet, data-driven models can make CII predictions with 85% accuracy — better than any physics-based model. With ‘noon report’ data , their accuracy can reach 99%.
That’s because AI isn’t bound by the limitations of the human mind. The “deep learning” systems used by modern AI can process more data than humans could in a lifetime, and draw conclusions in ways that are obscure to us but can be validated under real-world conditions. This year marks a turning point for maritime shipping. With the new IMO rules, carbon emissions will play a larger role than ever in business and operational decisions. The need for accurate modeling in this multi-trillion-dollar industry has never been greater. But also, ship owners and operators have more — and more accurate — options than ever.
About the author
Dylan Keil is the co-founder and CEO of Bearing, a Silicon Valley-based global startup powering the transition to green shipping. Bearing’s platform marries real-time vessel data with Artificial Intelligence to improve operational efficiency, provide competitive advantages, and reduce carbon emissions across the maritime shipping industry. Dylan is an experienced founder with deep expertise in building products that leverage real-world sensor data. Before founding Bearing, Dylan was the CEO and co-founder of Chronos, a startup that developed a leading contextual-awareness engine for mobile devices.